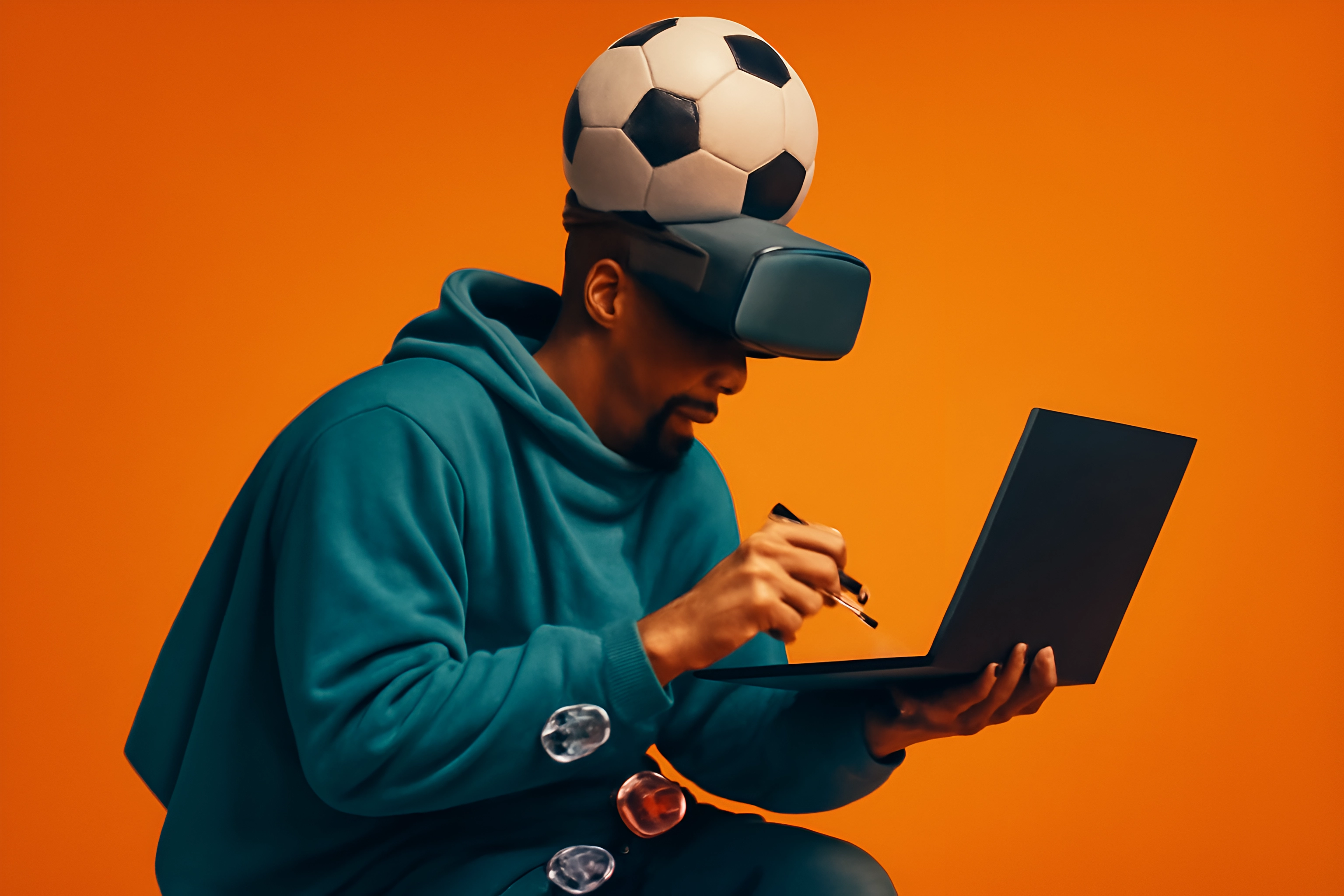
The world of sports betting has come a long way, hasn't it? Gone are the days when a simple gut feeling or a glance at the league table was enough. While basic knowledge certainly lays a foundation, true mastery in today's competitive landscape demands a much deeper, more analytical approach. Many bettors find themselves plateauing, relying on surface-level statistics or common knowledge, but the truth is, the edge is shrinking for those who don't adapt.
This is where the power of data comes into play. This post is your guide to unlocking that next level. We're going to explore how leveraging advanced sports analytics in betting can help you uncover hidden value, refine your betting models, and ultimately provide a significant advantage. We'll touch on key analytical concepts, discuss how to apply them practically, and even point you towards some valuable tools and resources. So, are you ready to transform your approach with advanced betting strategies? Let's dive in!
Beyond the Box Score: Why Advanced Analytics are Crucial for Modern Betting
We've all seen a box score, right? Points per game, win/loss records – these are the traditional stats we grew up with. While they offer a snapshot, they often don't tell the whole story, and that's a major limitation when serious money is on the line.
The real game-changer is how advanced analytics provide much deeper context and predictive power. Think about metrics like team efficiency, expected values based on play-by-play data, or how a team performs in very specific situations. This is where statistical betting analysis truly shines, moving beyond simple outcomes to understand why those outcomes occurred. As noted by experts in predictive analytics and gaming, these objective models help eliminate human bias and are key to how successful bettors gain an edge[3].
Ultimately, the goal for any serious bettor is to find an "edge" – a consistent advantage over the sportsbooks. This edge increasingly comes from superior information and, more importantly, the superior interpretation of that information. Companies like Stats Perform utilize vast amounts of data, combining real-time human analysis with AI to deliver granular metrics, turning raw numbers into actionable insights[4]. Their systems process an incredible 7.2 petabytes of historical data to help forecast outcomes with greater accuracy[4].
Key Pillars of Sports Analytics for Betting Success
So, what are these "advanced analytics" we're talking about? It's not just a single magic number. It's a collection of sophisticated metrics and methodologies that can be applied across various sports to give you a clearer picture. Let's break down some of the core concepts.
Player-Specific Advanced Metrics
Ever wondered how to truly quantify a single player's impact beyond just goals or points? That's where player-specific advanced metrics come in, and they are fantastic for spotting value. These stats aim to isolate an individual's contribution and efficiency.
Consider metrics like Player Efficiency Rating (PER) in basketball, Wins Above Replacement (WAR) in baseball, Expected Goals (xG) and Expected Assists (xA) in soccer, or Defense-adjusted Value Over Average (DVOA) in football. For instance, WAR in baseball quantifies a player's total value by combining their offensive, defensive, and baserunning contributions compared to a readily available "replacement-level" player[9]. Similarly, Expected Goals (xG) in soccer evaluates the quality of a shot based on numerous factors, using neural networks trained on over 100,000 shots to determine the probability of that shot resulting in a goal[11].
These metrics are powerful because they go beyond raw numbers to measure true impact and efficiency, often adjusting for context like opponent strength or park factors. How can you use this? This is your secret weapon for identifying undervalued or overvalued players, which is incredibly useful for player prop bets or even for assessing how a key player's presence (or absence) might affect the game line.
Team-Level Advanced Analytics
Just as with individual players, looking beyond a team's win-loss record is crucial. Team-level advanced analytics give us a much more accurate picture of a team's true strength and potential. These metrics help us understand how a team is winning or losing.
We're talking about stats like Pace (possessions per game), Offensive and Defensive Efficiency Ratings (points scored/allowed per 100 possessions), and even concepts like Pythagorean Wins. Pythagorean Wins, for example, estimates a team’s expected win total based on runs scored and allowed; the 2018 Seattle Mariners famously had an 89-73 record but a Pythagorean projection of only 77-85, signaling they were overperforming and likely due for regression[13]. Another powerful tool, especially in college basketball, is the KenPom rankings, which use an Adjusted Efficiency Margin (Net Rating) to measure team performance per 100 possessions, adjusted for opponent strength[20]. For example, Duke's 38.08 Net Rating in a given season indicated they were expected to beat an average D1 team by 38 points per 100 possessions[20].
By incorporating these kinds of analytics, you can better assess matchup dynamics and make more informed predictions about game outcomes. It’s about understanding the underlying process, not just the final score. This allows for a more nuanced view than simple standings can ever provide.
Situational & Contextual Analytics
The devil is often in the details, and that's certainly true in sports betting. Situational and contextual analytics involve dissecting performance based on specific circumstances, which can reveal patterns that aren't immediately obvious. This is where you can find some real hidden gems.
Think about how teams perform differently in various scenarios: Home/Away splits are a classic example, but we can go deeper. How does a team fare on the second night of a back-to-back? What's their record with less than two days of rest? How does the quantified impact of specific injuries (beyond just "Player X is out") affect team performance? Even weather conditions can be quantified and factored in. For instance, home and road splits in baseball can be significant, with some teams like the Braves historically showing large performance gaps depending on whether they play in their home park or away[15]. Another critical factor is Strength of Schedule (SOS), which helps differentiate team résumés by considering the quality of their opposition; the arithmetic mean SOS, averaging opponent ratings, is particularly useful for leagues like the NFL or NBA with relatively balanced schedules[14].
The beauty of this type of analysis is that data can reveal non-obvious patterns that the general betting public might overlook. By refining your predictions to factor in these often-overlooked variables, you can gain a significant advantage. It’s about adding layers of context to your analysis.
Introduction to Predictive Modeling Concepts
Now, let's touch upon something that sounds complex but is becoming increasingly accessible: predictive modeling. Don't worry, we're not diving into complex mathematical formulas here, just the core ideas behind these data-driven betting methods. These models are all about trying to forecast future outcomes.
You might hear terms like Regression Analysis, which is used to identify and quantify the relationships between different variables (e.g., how does a team's turnover rate correlate with its winning percentage?). Then there are Monte Carlo Simulations, a fascinating technique where a computer runs thousands, even millions, of simulated games based on input variables (player stats, team efficiencies, historical trends) to generate a range of possible outcomes and their probabilities[17]. For example, a simulation might predict a 60% chance of a home win[17]. Another popular approach involves Elo Ratings or Power Rankings, which are dynamic systems that assess team strength and update it after each game, considering factors like margin of victory and opponent strength; in many NFL Elo systems, a rating around 1505 is considered average[18]. These AI-driven forecasting models are becoming more prevalent in various fields, including sports.
The fundamental goal of these predictive sports modeling techniques is to forecast outcomes more accurately than the market or to identify discrepancies between their projections and the odds offered by sportsbooks. This is where you can start to build a truly systematic approach to finding value.
Applying Analytics: Actionable Data-Driven Betting Methods
Understanding these analytical concepts is one thing, but how do we actually use them to make smarter bets? That's the million-dollar question, isn't it? Let's explore some actionable ways to integrate these data-driven betting methods into your routine.
Identifying Value Bets with Statistical Discrepancies
This is the holy grail for many analytical bettors: finding value. The core idea is to compare your own analytical assessment of a game or event (perhaps derived from your own research or a model's output) to the odds offered by the sportsbooks. If your analysis suggests a higher probability of an outcome than the odds imply, you've potentially found a value bet.
This brings us to the concept of Expected Value, or EV. As explained in resources on Expected Value in betting, a positive Expected Value (EV+) bet is one where your calculated probability of winning is greater than the sportsbook's implied probability[19]. For example, if your model gives a team a 55% chance to win, but the odds imply only a 50% chance, that 5% difference represents your edge, leading to a +EV situation[19]. Consistently identifying and betting on +EV opportunities is a cornerstone of long-term profitability in sports betting.
It’s about finding those spots where the market might be undervaluing a team or player based on deeper statistical insights. This is where betting value with analytics truly comes to life, turning your hard analytical work into potential profit.
Leveraging Analytics for Prop Betting
Player proposition bets, or prop bets, are an area where analytics can really shine. Instead of just betting on the game outcome, you're wagering on an individual player's performance – like points scored, yards gained, number of shots, and so on. This is where those player-specific advanced metrics we discussed earlier become incredibly powerful.
Think about it: if you're using metrics like xG for soccer players or WAR for baseball players, you have a much more nuanced understanding of a player's true potential and recent form than someone just looking at basic goals or hits. You can compare a player's advanced statistical profile against the lines offered by sportsbooks for their props. For example, if a soccer player has a high xG but hasn't scored in a few games, they might be due for a goal, and their "to score anytime" prop could offer value.
By diving deep into player-specific data, you can often find edges in these more niche markets that others might overlook. This requires a bit more detailed research, but the payoff can be well worth the effort for those willing to dig into the numbers.
Enhancing Live Betting Decisions with Real-Time Data
Live betting, or in-play betting, has exploded in popularity, and it's an area where quick, data-informed decisions can make a huge difference. If you have access to in-game analytics or have robust pre-game models, you can use this information to make smarter live betting adjustments as the game unfolds.
Imagine a basketball game where one team is on a run. Is it just a hot streak, or do underlying efficiency metrics suggest this performance is sustainable or, conversely, likely to regress? Understanding game flow and momentum through a statistical lens, rather than just "feel," can help you identify valuable live betting opportunities, perhaps on point spreads, totals, or the next team to score. Some advanced data providers even offer real-time predictive adjustments that can feed into live betting models.
The key here is to have a plan and understand what statistical indicators to look for that might signal a shift in the game's dynamics. This allows you to react intelligently to the ebb and flow of live action, rather than betting impulsively.
Building a Basic Analytical Framework/Spreadsheet
Feeling a bit overwhelmed by all these metrics and models? Don't be! You don't need to become a data scientist overnight to start benefiting from analytics. A great way to begin is by building a basic analytical framework, perhaps even just a simple spreadsheet.
Start by identifying a few key data points or advanced metrics that resonate with you for a particular sport. Begin tracking this data for upcoming games, perhaps developing your own simple rating system or comparative analysis. For example, you could track team offensive/defensive efficiencies, pace, and recent form against the spread. Many bettors find that using essential digital marketing tools for modern marketers, like spreadsheets, can be surprisingly effective for organizing sports data and spotting trends.
The most important thing is to focus on the process and commit to continuous refinement. Your initial framework won't be perfect, but as you gather more data and learn what works, you can gradually improve your models and your understanding. This hands-on approach is one of the best ways to learn.
Tools and Resources for the Analytical Bettor
So, where can you find all this juicy data and what tools can help you make sense of it? Fortunately, there's a growing ecosystem of resources available for the analytically-minded bettor. It's all about knowing where to look.
You can often find reliable advanced stats on official league websites, but there are also reputable third-party providers that specialize in sports data. Companies like Opta (by Stats Perform) are industry leaders, providing incredibly granular data, such as their F24 feed which offers XY coordinates for soccer passes and shots, enabling deep analysis of possession networks[2]. Their data, including Expected Goals (F73) and even off-ball run metrics, powers features on major betting platforms and is invaluable for building sophisticated models[2]. Another excellent resource, particularly for soccer, is StatsBomb, known for its detailed event data and insightful visualizations like player radar charts that cover over 140 leagues[6]. For college basketball, KenPom is a household name, and for football, Football Outsiders offers deep DVOA metrics.
In terms of software, you can start with familiar tools like Excel or Google Sheets for basic data tracking and analysis. For those with more technical skills or ambitions, learning programming languages like R or Python can unlock powerful data manipulation and modeling capabilities. Don't forget about online communities and forums either; these can be great places to discuss analytical approaches, share insights, and learn from fellow bettors. While we don't have specific reviews on SportsBettinger right now, exploring these types of technical optimization tools for digital marketers can sometimes provide inspiration for data handling.
The Caveats: Limitations and Responsible Application of Analytics
Now, before we get completely carried away by the allure of data, it's crucial to inject a healthy dose of realism. Analytics are an incredibly powerful tool, but they are not a crystal ball. There are limitations and important considerations to keep in mind.
Firstly, sports are played by humans, not robots, and there will always be unquantifiable factors that data alone can't capture. Things like team motivation, sudden shifts in momentum, coaching genius (or blunders!), and sheer luck will always play a role. Moreover, the quality of data and the sample size you're working with are critical; small sample sizes can lead to misleading conclusions. There's also the risk of "overfitting" models, where your model becomes too closely tailored to past data and loses its predictive power for future events. For example, while data on rest days is important – WHOOP data shows athletes with low strain on rest days are 2.3x less likely to underperform the next day[16] – it doesn't capture every nuance of individual player recovery.
Most importantly, analytics should always complement, not replace, sound betting principles. This means disciplined bankroll management, understanding value, and always gambling responsibly. No analytical model, no matter how sophisticated, can guarantee wins. (If SportsBettinger had articles on bankroll management or responsible gambling, this would be a perfect spot to link them!)
Conclusion: Elevating Your Betting Game with an Analytical Edge
Wow, we've covered a lot of ground, haven't we? From understanding the limitations of basic stats to exploring the depths of player-specific metrics, team efficiencies, and even predictive modeling, it's clear that integrating advanced sports analytics in betting can truly elevate your game. It’s about moving from hunches to informed hypotheses.
Remember, this is a journey of continuous learning and refinement. The world of sports analytics is constantly evolving, with new metrics and methodologies emerging all the time. Don't feel like you need to master everything at once. The best approach is to start small, pick a sport or a specific metric that interests you, and gradually build your analytical toolkit from there.
As the sports betting landscape becomes more sophisticated, mastering analytics is becoming increasingly key to achieving long-term success and maintaining that coveted edge. By embracing these advanced betting strategies, you're equipping yourself with the tools to make smarter, more data-driven decisions.